DeepSeek-R1: Redefining Reasoning in AI
Published on January 28, 2025
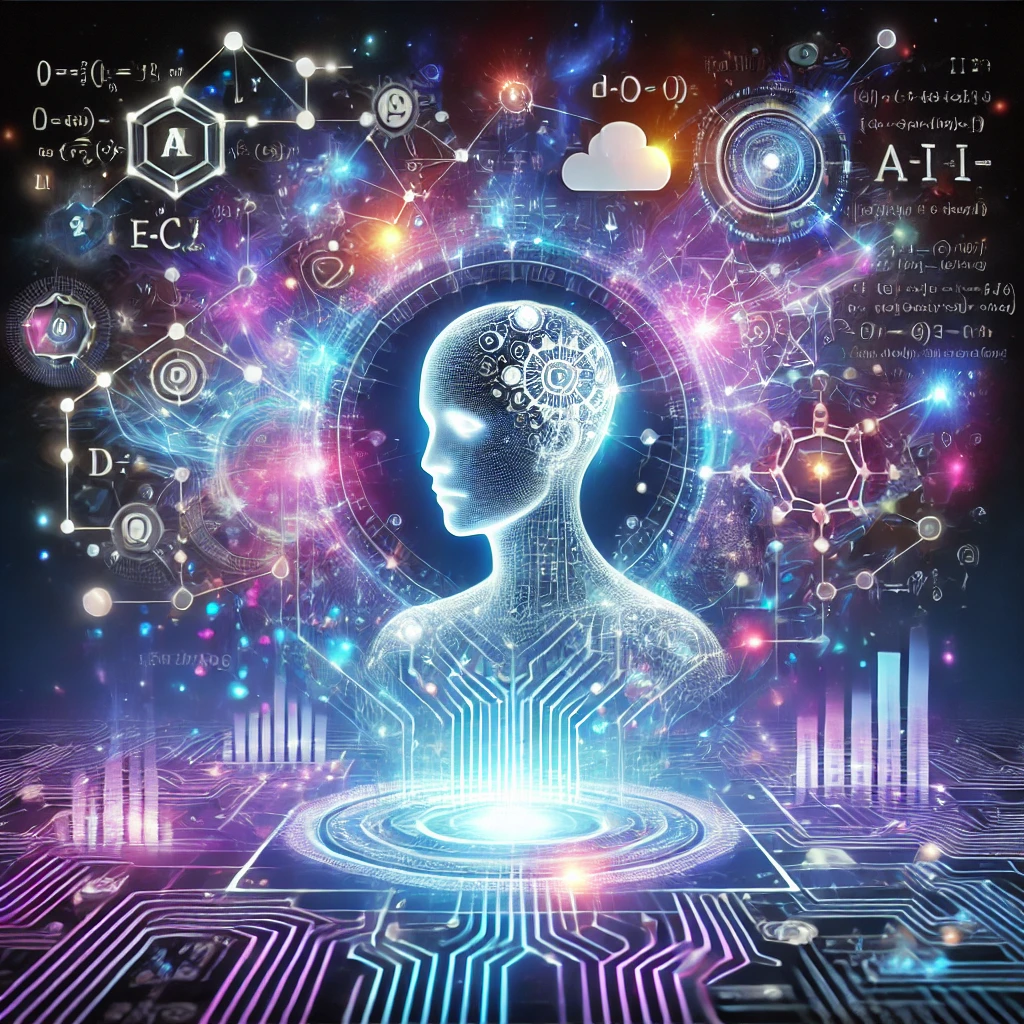
Reinforcement Learning Without a Safety Net
DeepSeek-R1 represents a bold departure from traditional AI training methodologies. By relying on pure reinforcement learning (RL) without the safety net of supervised fine-tuning (SFT), the researchers behind DeepSeek-R1-Zero unlocked reasoning behaviors that rivaled OpenAI’s state-of-the-art models. As the white paper describes:
"DeepSeek-R1-Zero demonstrates capabilities such as self-verification, reflection, and generating long Chains of Thought (CoT), marking a significant milestone for the research community." (DeepSeek White Paper)
This approach eliminates the reliance on vast, curated datasets, allowing the model to "learn how to think" rather than being told what to think.
From Aha Moments to Autonomous Reasoning
Perhaps the most captivating insight from DeepSeek-R1-Zero is the emergence of unexpected reasoning behaviors. These "aha moments" illustrate the raw potential of RL-driven AI:
"The model learns to allocate more thinking time to a problem by reevaluating its initial approach, showcasing self-evolution and sophisticated problem-solving strategies."
By simply providing incentives for better outcomes, DeepSeek-R1-Zero autonomously developed advanced reasoning strategies, signaling a new era of adaptive AI development.
DeepSeek-R1: Bridging Performance and Readability
Despite its breakthroughs, DeepSeek-R1-Zero faced challenges such as poor readability and language mixing. Enter DeepSeek-R1—a model that integrates multi-stage RL with cold-start data to address these issues. The result is a system capable of outperforming OpenAI’s o1-1217 in key benchmarks, including a 97.3% accuracy on MATH-500 and an impressive 90.8% on MMLU.
"DeepSeek-R1 achieves performance on par with OpenAI-o1-1217 on reasoning tasks, leveraging structured cold-start data to improve both coherence and performance."
This balance between accuracy and accessibility makes DeepSeek-R1 a game-changer for both researchers and developers.
Smaller Models, Bigger Impact
A critical breakthrough from DeepSeek is the distillation of its reasoning capabilities into smaller, dense models. This technique demonstrates that compact AI systems can inherit the strengths of their larger counterparts:
"The distilled 14B model outperforms state-of-the-art open-source QwQ-32B-Preview by a large margin, setting a new record on reasoning benchmarks among dense models."
This innovation democratizes access to high-performance AI, enabling more organizations to leverage advanced reasoning models without requiring extensive computational resources.
Challenges and the Road Ahead
The white paper also highlights areas for improvement, such as prompt engineering, multilingual coherence, and scaling reinforcement learning for software engineering tasks. These challenges underscore the ongoing evolution of DeepSeek-R1 and its potential future iterations:
"DeepSeek-R1’s sensitivity to prompts indicates that zero-shot settings yield better performance, but addressing this limitation will further enhance its usability in diverse scenarios."
As the research team explores these avenues, the implications for AI-driven reasoning in real-world applications are boundless.
Final Thoughts: The DeepSeek Legacy
DeepSeek-R1 is more than just a model—it’s a blueprint for the future of reasoning AI. By shifting the focus from raw computational power to intelligent training techniques, DeepSeek is not only redefining what AI can achieve but also who can achieve it.
Explore these advancements and see how they could transform your projects. Visit AIRWEB to learn how this new era in AI is driving innovation for businesses and developers alike.