Exploring Best Practices in Retrieval-Augmented Generation (RAG)
Published on December 2, 2024
🤖 Get AI Summary of this Post:
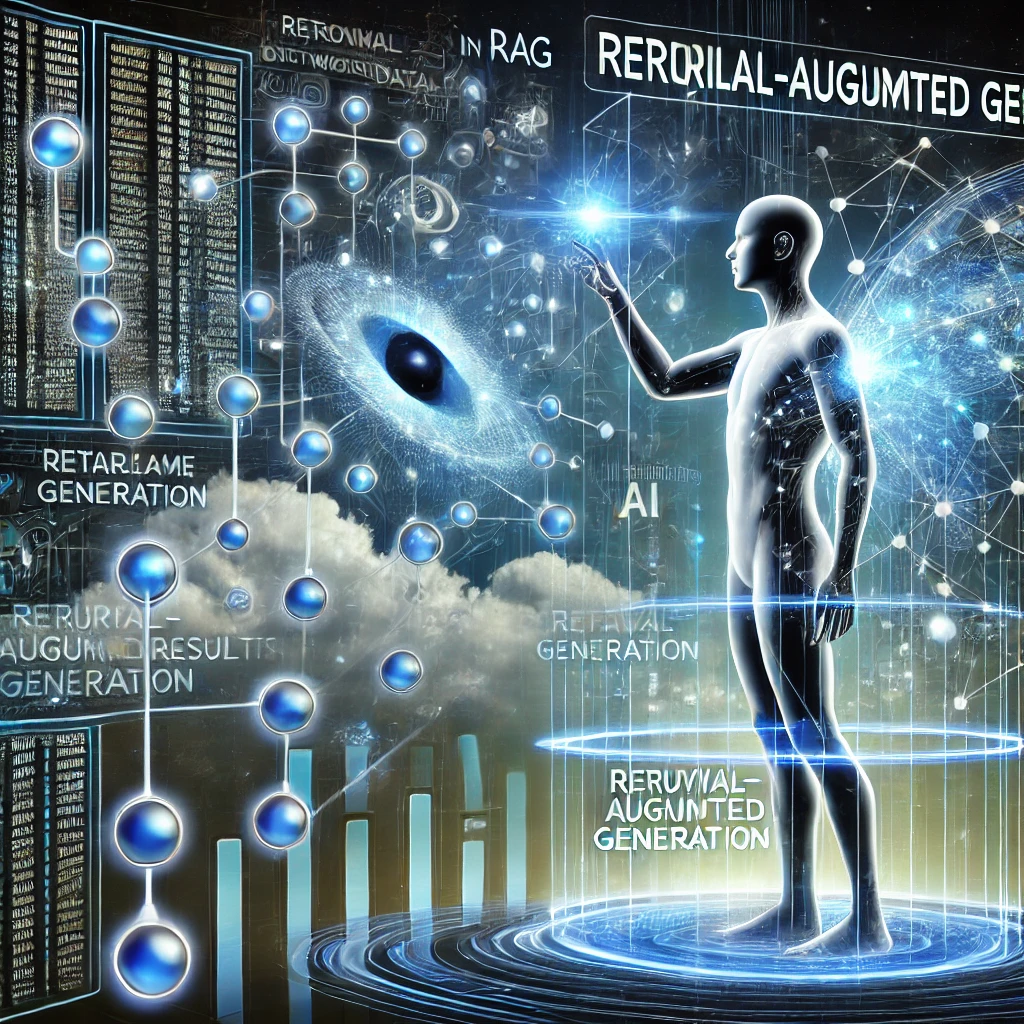
Retrieval-Augmented Generation (RAG) has emerged as a transformative approach to combining the strengths of pretraining with real-time retrieval capabilities. A recent study titled "Searching for Best Practices in Retrieval-Augmented Generation" provides groundbreaking insights into optimizing RAG workflows. Presented at the 2024 EMNLP Conference, this research by Xiaohua Wang et al. systematically explores optimal strategies for implementing RAG in diverse contexts.
The RAG workflow integrates various steps, including query classification, document retrieval, reranking, repacking, and summarization. Each step involves choices that significantly impact performance, efficiency, and response quality. This study is pivotal in addressing challenges like complex implementation and response latency, offering a detailed roadmap for achieving balanced and effective RAG systems.
Key Findings in RAG Optimization
1. Query Classification: Efficiently determines whether retrieval is necessary for a given query, saving computational resources and time. This step alone improved response latency by 29% while enhancing accuracy.
2. Hybrid Retrieval with HyDE: Combines dense retrieval methods with BM25 for complementary strengths. This approach maximizes semantic understanding and precision, achieving the highest RAG scores in experiments.
3. MonoT5 Reranking: A standout for enhancing document relevance, significantly improving the system's response quality.
4. Repacking and Summarization: The "reverse" repacking strategy and Recomp summarization ensure the most relevant information is prioritized and concisely presented, boosting overall system utility.
"This research redefines best practices in RAG, delivering a practical framework for maximizing efficiency and efficacy across varied domains."
Applications and Implications
The implications of this research span multiple industries. From enhancing medical QA systems to streamlining customer service interactions, the optimized RAG workflow addresses critical needs for accuracy and scalability. Furthermore, the study introduces multimodal extensions, including image-text retrieval, which expands RAG's applicability to visual and multimodal content generation.
At Reality Border, these advancements inspire us to push the boundaries of AI solutions. Integrating such best practices into platforms like Airweb.ai ensures our clients benefit from cutting-edge, efficient, and reliable AI-driven interactions.
Shaping the Future of AI
For detailed insights into the RAG workflow and the recommended best practices, access the full paper on the ACL Anthology. Join us in exploring how innovations in AI can redefine efficiency, scalability, and real-time intelligence for businesses worldwide.